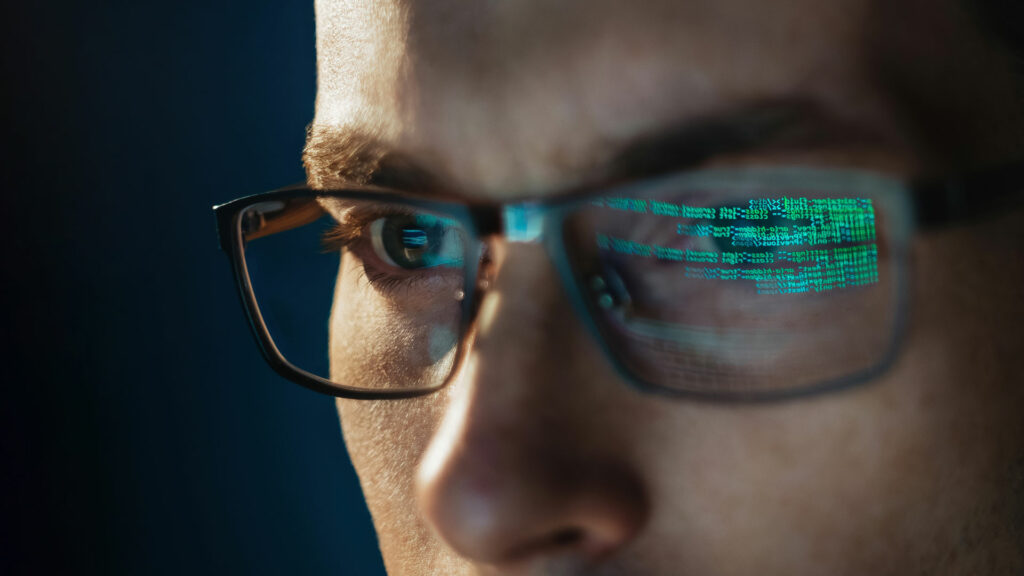
In developing a new metric, known as Conceptual Consistency, SRI researchers measure how much AI truly knows.
Computers that see. Chatbots that chat. Algorithms that paint on command. The world is finally getting a glimpse of the true promise of artificial intelligence (AI). And yet an argument rages as to whether these applications are truly intelligent. It is a question that goes to the very heart of what it means to be a human beingācomprehending of the world around us; able to create ideas, words, and other new things; and possessing of self-awareness.
Now a team of researchers led by SRI Internationalās Ajay Divakaran, technical director of the Vision and Learning Laboratory at SRIās Center for Vision Technologies, has set out to answer a provocative question: How much does AI really āunderstandā about the world? Divakaran, SRI colleagues Michael Cogswell and Yunye Gong, former intern Pritish Sahu, and Professor Yogesh Rawat and his doctoral student Madeleine Schiappa at the University of Central Florida have developed a way to calculate just how much artificial intelligence knows. They call it conceptual consistency.
āDeep learning models, like ChatGPT, DALL-E, and others, have demonstrated fairly remarkable performance in many humanlike tasks, but it is not clear if they do so by mere rote memory or possess true conceptual models of the way the world works,ā Divakaran says.
He provides an example from one of the teamās papers of a visual and language (V+L) model trained to evaluate and describe images. A conceptually consistent model should know that the description āsnow garnished with a manā is not only implausible but impossible. By the same token, Divakaran says, a similar model should be able to positively assert that a chair is not just a chair but a beach chair by taking contextual clues from the imageāfor instance, that the chair in question is situated on a beach.
Seemingly simple but creative leaps in logic and reasoning like these are hallmarks of human intelligence, Divakaran says, and are critical to the sort of truly intelligent AI used in life-and-death applications like autonomous cars and airplanes. In these uses, AI must understand the world rather than fall back on mere memory. The researchers hope it can help developers of AI improve the reliability of their applications.
āWe have developed a way to test this key distinction, and we can use it to evaluate when we can have faith in AIās capabilities and when we need to be more skeptical of AI and more conservative in our use of these still-new technologies,ā he explains.
Conceptual consistency works whether the output being judged is language, as with ChatGPT, or images, as with DALL-E and other algorithms that can āseeā and identify objects in photographs. Divakaran and colleagues refer to these as multimodal models. A computer vision algorithm used in an autonomous vehicle must be able to see objects in the world, know what they are, and reason about how to respond to those objects.
āIn its most basic level, conceptual consistency measures whether AIās knowledge of relevant background information is consistent with its ability to answer follow-on questions correctly,ā Divakaran says. āConceptual consistency measures AIās depth of understanding.ā
In one paper, Divakaran and his co-authors provide the example query, āIs a mountain tall?ā A large language model (LLM) is likely to answer correctly, with a simple āYes.ā While that is all well and good, it is hardly remarkable, Divakaran would argue. Whatās more important, and more indicative of true intelligence, is the generalizability of the modelās understanding about mountainsāits conceptual consistency. A conceptually consistent model should also be able to answer more difficult queries about mountains correctly. But often the deeper one probes, the less conceptually consistent the models become.
The great fear and a still-open question, skeptics argue, is whether an LLM can answer only from its existing knowledge base and therefore cannot produce the sort of creative or tangential leaps of the best human minds.
āLLMsā memory is limited to the data they have at their disposal and is therefore only mimicking the data used to train them, using probability to assemble words and ideas through pattern recognition in ways that other humans have in the past,ā Divakaran explains.
To put it simply, AI does not have a mind of its ownāit is simply repeating or perhaps reorganizing what other human minds have already produced. By measuring background knowledge and predicting a modelās ability to answer questions correctly on a given topic, the SRI team computes conceptual consistency to quantify when a modelās knowledge of relevant background is consistent with its ability to perform a given task.
In experiments, Divakaran and colleagues have arrived at several interesting conclusions. A modelās knowledge of background information can be used to predict when it will answer questions correctly, and conceptual consistency generally grows with the scale of the model. āBigger models are not just more accurate, but also more consistent,ā Divakaran and co-authors wrote in one of their recent papers. GPT-3, the LLM behind ChatGPT, does show a moderate amount of conceptual consistency. But, by the same token, multimodal models have not been investigated rigorously.
āAt the very least, conceptual consistency can help us know when itās safe to trust AI and when a go-slow approach is warranted,ā Divakaran says.