Simplifying the process of detecting altered and tampered videos.
CHALLENGE AND GOAL
As powerful video editing software tools become more commonplace, it has become easier for anyone to tamper with content. Rapidly developing consumer applications have made it possible for almost anyone to create synthesized speech and synthesized video of a person talking.
SOLUTION AND OUTCOME
SRI International researchers are working with the University of Amsterdam and Idiap Research Institute to develop new techniques for detecting videos that have been altered. The Spotting Audio-Visual Inconsistencies (SAVI) techniques, developed by SRI, detect tampered videos by identifying discrepancies between the audio and visual tracks.

In today’s connected society, it’s impossible to reasonably trust anything that is seen online. Advances in technology have created a world where anyone can tamper with multimedia content to make it appear that an individual did or said something, when in reality it never occurred.
To help combat this issue, researchers at SRI are working to develop technology that enables the public to detect altered or tampered video. The techniques, referred to as Spotting Audio-Visual Inconsistencies (SAVI) can detect when lip synchronization is a little off or if there is an unexplained visual “jerk” in the video.
It can also flag a video as possibly tampered if the visual scene is outdoors, but analysis of the reverberation properties of the audio track indicates the recording was done in a small room.

This video shows how the SAVI system detects speaker inconsistencies. First, the system detects the person’s face, tracks it throughout the video clip and verifies it is the same person for the entire clip. It then detects when she is likely to be speaking by tracking when she is moving her mouth appropriately.
The system analyzes the audio track, segmenting it into different speakers. As shown in the image below, the system detects two speakers: one represented by the dark blue horizontal line and one by the light blue line. Since there are two audible speakers and only one visual person, the system flags the segments associated with the second speaker as potentially tampered – represented by the horizontal red line.
The system also detects lip synch inconsistency by comparing visual motion features with audio features. It computes the visual features by detecting the person’s face, using OpenPose to detect and track face landmarks, and computing a spatiotemporal characterization of mouth motion, as seen below.
The SAVI system combines these findings with Mel-frequency cepstral coefficients (MFCC) features of the audio track to classify 2-second video clips as either good lip synchronization or bad, based on a large training set of audiovisual feature vectors. The system marks inconsistencies in red and consistencies in green along the horizontal line at the bottom of the image.
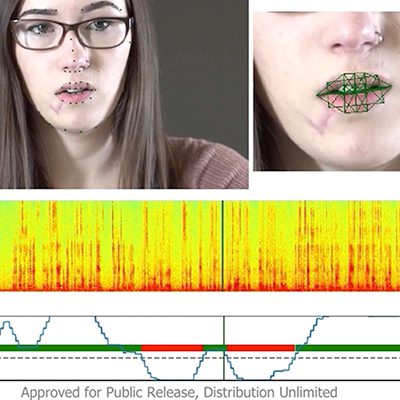