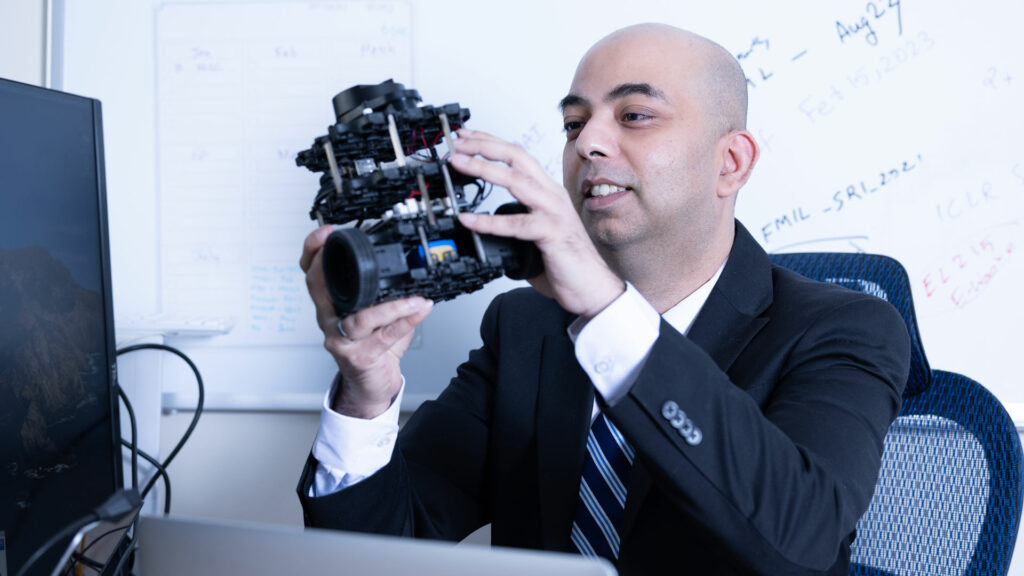
SRI is developing a hybrid AI to improve human performance by combining two AI methods to continuously learn and adapt to rapidly evolving novel situations.
Increasingly, artificial intelligence (AI) is being deployed across several civilian and military applicationsâto fight forest fires and cyberattacks, and on battlefieldsâthat require complex situational comprehension to help humans make faster, smarter decisions.
The high-stakes nature of these situations requires AI solutions to be robust to data they have never seen before, not even in training. Safety-critical decision-making in these situations requires humanlike vision, hearing, and reasoning capabilities to deal with rapidly evolving events. It also needs to be transparent so humans understand how and why the AI arrived at its recommendations. Unfortunately, these parameters are beyond the reach of current AI methods.
In response, the Defense Advanced Research Projects Agency (DARPA) has started a new research programâAssured Neuro-Symbolic Learning and Reasoning (ANSR)âto motivate new thinking and approaches that can help assure autonomous systems will operate safely and perform as intended. The program includes a research collaborative led by SRI and partners at leading AI programs at three top research universitiesâCarnegie Mellon University, the University of California at Los Angeles, and the U.S. Military Academy.
The SRI-led team is developing a hybrid AI to improve human performance by combining two AI methodsâsymbolic deductive reasoning and data-driven deep learningâto continuously learn and adapt to never-before-seen situations. They call their hybrid approach TrinityAIâTrustworthy, Resilient, and Interpretable AI.
âWe are combining the two leading and complementary approaches to AIâdeep neural networks based on large language models and symbolic reasoningâto produce a hybrid AI system that can be trusted to operate in the presence of novel inputs outside its training, is resilient to adversarial perturbations, and improves interpretability by providing the rationale for its decisions,â said Susmit Jha, a technical director at SRI and principal investigator on the TrinityAI ANSR project.
The mindâs eye
The teamâs approach is based on âPredictive Processing: A Theory of Mindââa model that explains how the human brain evaluates the world around it and makes decisions. In predictive processing, the human brain is constantly sizing up the world and generating a holistic mental model of the way things work.
Then, based on new dataâsensory input from the eyes and ears, mainlyâthe brain continuously updates that model. In essence, the brain generates hypothetical predictions about the world based on existing information and measures those expectations against real sensory inputs.
âWe continuously create and maintain predictive models of the world and then interpret our observations in the context of these models, making the inferences we draw stronger,â Jha says. âWith TrinityAI, we are working to develop a hybrid AI system that does the same to improve confidence in AI-supported decision-making.â
Imagine trying to use AI to operate in a complex environment such as a battlefield, where civilian and military personnel, structures, and equipment are intermixedâand often camouflaged. A trustworthy AI would be able to recognize not just friend from foe but also neutral entities. It could also size up the operating environment and discern safer paths of passage from more dangerous ones.
ANSR will investigate these hybrid architectures and feed them diverse data about the real world, then use both neural and symbolic learning to make assessments of complex situations. Neural networking takes a more âhuman brainâlikeâ approach to learning, running through permutations in search of patterns in words and images to produce insights that might be imperceptible to even highly trained humans. Symbolic learning, on the other hand, is more deductive.
A matter of trust
The ultimate measuring stick of success is the degree of trust TrinityAIâs human collaborators have in its decision-making powers. The project team defines âtrustworthyâ in several ways. First and foremost, the system must be impenetrable to adversarial manipulation.
It also must be able to correctly analyze different types of inputsâvisual, aural, spoken and written words, and more. Finally, the system must return predictable results that can be evaluated for accuracy. TrinityAI must be able to tell decision-makers how confident it is in its own predictions.
Thatâs a tall order, but Jha says the assembled team had already made progress toward these goals in prior research in the DARPA Assured Autonomy program, where he led an SRI team with MIT and Caltech as subcontractors.
âTrinityAI will advance existing approaches in three ways: improving trust, interpretability, and, ultimately, the systemâs robustness, even in novel situations with limited data,â Jha says.
âThis hybrid AI approach inspired by the theory of mind will pay big dividends in the trustworthiness of AI and its responsible adoption in safety-critical applications.â