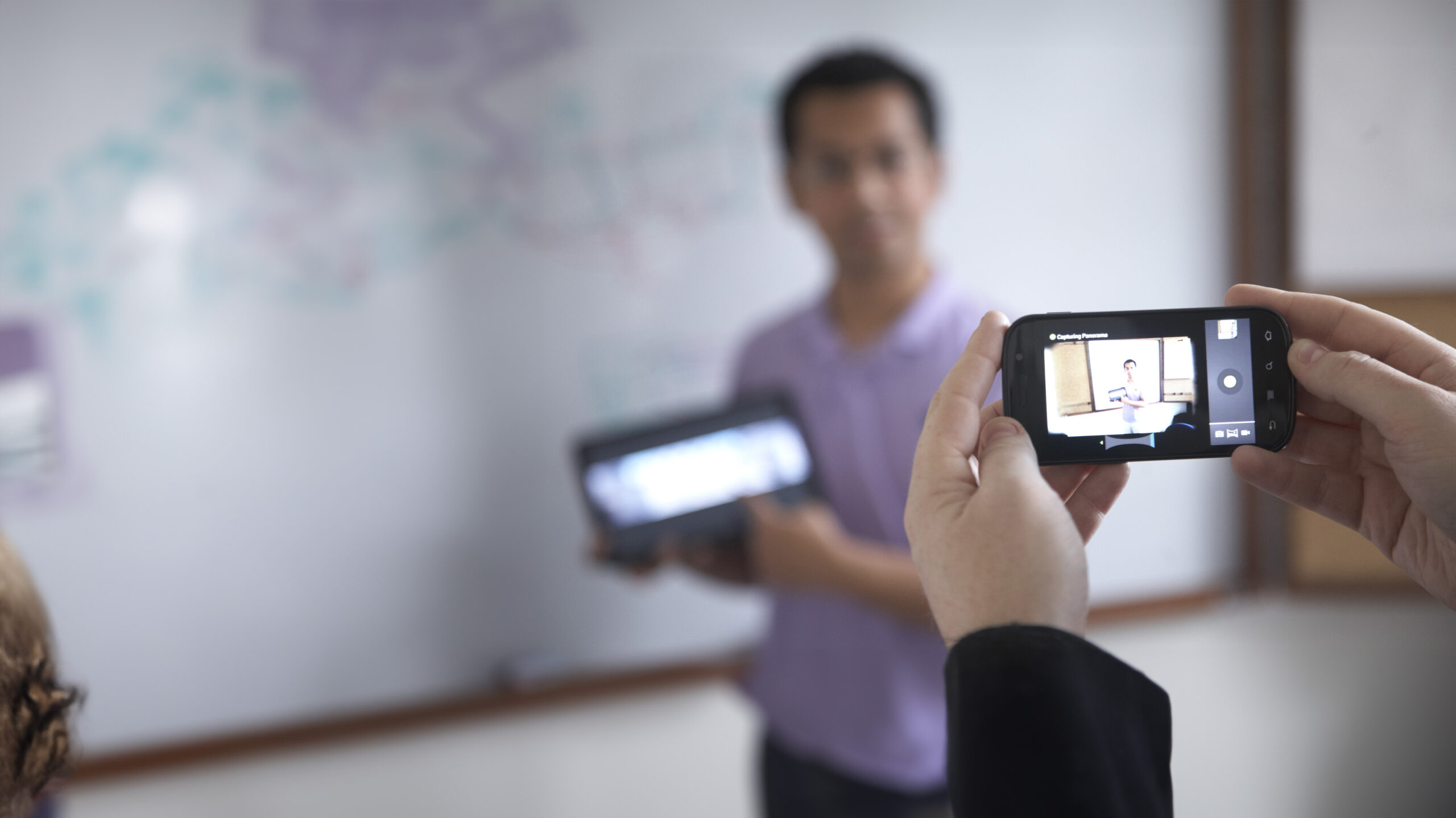
Center for vision technologies
Fundamental computer vision solutions based on leading-edge technologies, leveraging a variety of sensors and computation platforms
CVT does both early-stage research and developmental work to build prototype solutions that impact government and commercial markets, including defense, healthcare, automotive and more. Numerous companies have been spun-off from CVT technology successes.
The Center for Vision Technologies (CVT) develops and applies its algorithms and hardware to be able to see better with computational sensing, understand the scene using 2D/3D reasoning, understand and interact with humans using interactive intelligent systems, support teamwork through collaborative autonomy, mine big data with multi-modal data analytics and continuously learn through machine learning.
Recent developments from CVT include core machine learning algorithms in various areas such as learning with fewer labels, predictive machine learning for handling surprise and novel situations, lifelong learning, reinforcement learning using semantics and robust/explainable artificial intelligence.
SmartVision imaging systems use semantic processing/multi-modal sensing and embedded low-power processing for machine learning to automatically adapt and capture good quality imagery and information streams in challenging and degraded visual environments.
Multi-sensor navigation systems are used for wide-area augmented reality and provide GPS-denied localization for humans and mobile platforms operating in air, ground, naval, and subterranean environments. CVT has extended its navigation and 3D modeling work to include semantic reasoning, making it more robust to changes in the scene. Collaborative autonomy systems can use semantic reasoning, enabling platforms to efficiently exchange dynamic scene information with each other and allow a single user to control many robotic platforms using high-level directives.
Human behavior understanding is used to assess human state and emotions (e.g., in the Toyota 2020 concept car) and to build full-body, multi-modal (speech, gesture, gaze, etc.) human-computer interaction systems.
Multi-modal data analytics systems are used for fine-grain object recognition, activity, and change detection and search in cluttered environments.
Our work
-
SRI to develop high throughput reconfigurable radio frequency processors
Radio processors will be needed in communication environments where static solutions often fail.
-
SRI squeezes computer processors between pixels of an image sensor
The microscale computers will speed the processing of volumes of image data generated by autonomous vehicles, robots, and other devices that “see.”
-
SRI researchers seek to help AI chatbots deliver more reliable responses
Drawing inspiration from human learning, researchers are guiding chatbots to go beyond mere memorization of statistical patterns to understanding of context.
Core technologies and applications
SRI’s Center for Vision Technologies (CVT) tackles data acquisition and exploitation challenges across a broad range of applications and industries. Our researchers work in cross-disciplinary teams, including robotics and artificial intelligence, to advance, combine and customize technologies in areas including computational sensing, 2D-3D reasoning, collaborative autonomy, human behavior modeling, vision analytics, and machine learning.
Publications
by research area
Computational sensing and low-power processing
-
Low-Power In-Pixel Computing with Current-Modulated Switched Capacitors
We present a scalable in-pixel processing architecture that can reduce the data throughput by 10X and consume less than 30 mW per megapixel at the imager frontend.
2d 3d reasoning and augmented reality
-
Vision based Navigation using Cross-View Geo-registration for Outdoor Augmented Reality and Navigation Applications
In this work, we present a new vision-based cross-view geo-localization solution matching camera images to a 2D satellite/ overhead reference image database. We present solutions for both coarse search for…
Collaborative human-robot autonomy
-
Ranging-Aided Ground Robot Navigation Using UWB Nodes at Unknown Locations
This paper describes a new ranging-aided navigation approach that does not require the locations of ranging radios.
Human behavior modeling
-
Towards Understanding Confusion and Affective States Under Communication Failures in Voice-Based Human-Machine Interaction
We present a series of two studies conducted to understand user’s affective states during voice-based human-machine interactions.
Multi-modal data analytics
-
Time-Space Processing for Small Ship Detection in SAR
This paper presents a new 3D time-space detector for small ships in single look complex (SLC) synthetic aperture radar (SAR) imagery, optimized for small targets around 5-15 m long that…
Machine learning
-
Unsupervised Domain Adaptation for Semantic Segmentation with Pseudo Label Self-Refinement
We propose an auxiliary pseudo-label refinement network (PRN) for online refining of the pseudo labels and also localizing the pixels whose predicted labels are likely to be noisy.
Publications
-
Unsupervised Domain Adaptation for Semantic Segmentation with Pseudo Label Self-Refinement
We propose an auxiliary pseudo-label refinement network (PRN) for online refining of the pseudo labels and also localizing the pixels whose predicted labels are likely to be noisy.
-
C-SFDA: A Curriculum Learning Aided Self-Training Framework for Efficient Source Free Domain Adaptation
We propose C-SFDA, a curriculum learning aided self-training framework for SFDA that adapts efficiently and reliably to changes across domains based on selective pseudo-labeling. Specifically, we employ a curriculum learning…
-
Night-Time GPS-Denied Navigation and Situational Understanding Using Vision-Enhanced Low-Light Imager
In this presentation, we describe and demonstrate a novel vision-enhanced low-light imager system to provide GPS-denied navigation and ML-based visual scene understanding capabilities for both day and night operations.
Computer vision leadership
-
William Mark
Senior Technology Advisor, Commercialization
-
Rakesh “Teddy” Kumar
Vice President, Information and Computing Sciences and Director, Center for Vision Technologies
Our team
-
Rakesh “Teddy” Kumar
Vice President, Information and Computing Sciences and Director, Center for Vision Technologies
-
Supun Samarasekera
Senior Technical Director, Vision and Robotics Laboratory, Center for Vision Technologies
-
Michael Piacentino
Senior Technical Director, Vision Systems Laboratory, Center for Vision Technologies
-
Han-Pang Chiu
Technical Director, Vision and Robotics Laboratory, Center for Vision Technologies
-
Bogdan Matei
Technical Director, Vision and Robotics Laboratory, Center for Vision Technologies